Introduction
The global transition towards renewable energy is not merely a choice but a necessity to combat climate change and ensure energy sustainability. However, the intermittent nature of renewable energy sources like solar and wind poses a significant challenge. This is where Artificial Intelligence (AI) and Machine Learning (ML) step in, providing solutions to optimize renewable energy systems. Through predictive maintenance, energy forecasting, automated energy trading, and enhanced design and deployment, AI and ML are proving to be indispensable tools in the renewable energy sector.
I. Understanding the Basics
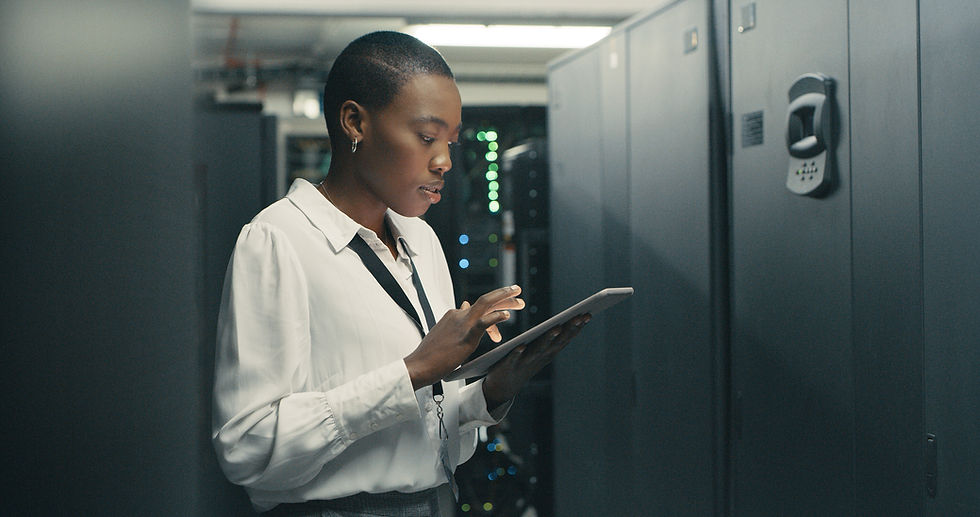
A. Definition and Brief Overview of AI and ML
Artificial Intelligence (AI) is a broad field of computer science focused on creating smart machines capable of performing tasks that typically require human intelligence. Machine Learning (ML), a subset of AI, involves the development of algorithms that can learn from and perform predictive analysis or other kinds of decision-making based on data.
B. Introduction to Renewable Energy Systems
Renewable energy systems harness natural resources such as sunlight, wind, and water to generate electricity. The primary types include solar, wind, hydroelectric, geothermal, and biomass energy systems, each with distinct characteristics and technological requirements.
II. Predictive Maintenance and Monitoring
A. Description of Predictive Maintenance
Predictive maintenance utilizes AI and ML algorithms to anticipate equipment failures and optimize maintenance schedules, thus reducing downtime and operational costs. By analyzing vast datasets, these algorithms can predict when components like wind turbine blades or solar panels are likely to fail or require maintenance.
B. Case Studies
Various renewable energy facilities globally have integrated AI-driven predictive maintenance to enhance their operations. For instance, wind farms use AI to monitor the condition of turbines continuously, thus enabling timely maintenance and preventing costly breakdowns.
C. Benefits
The implementation of predictive maintenance results in cost reduction, efficiency improvement, and extended equipment lifespan, thus contributing to the overall profitability and sustainability of renewable energy projects.
III. Energy Forecasting and Optimization
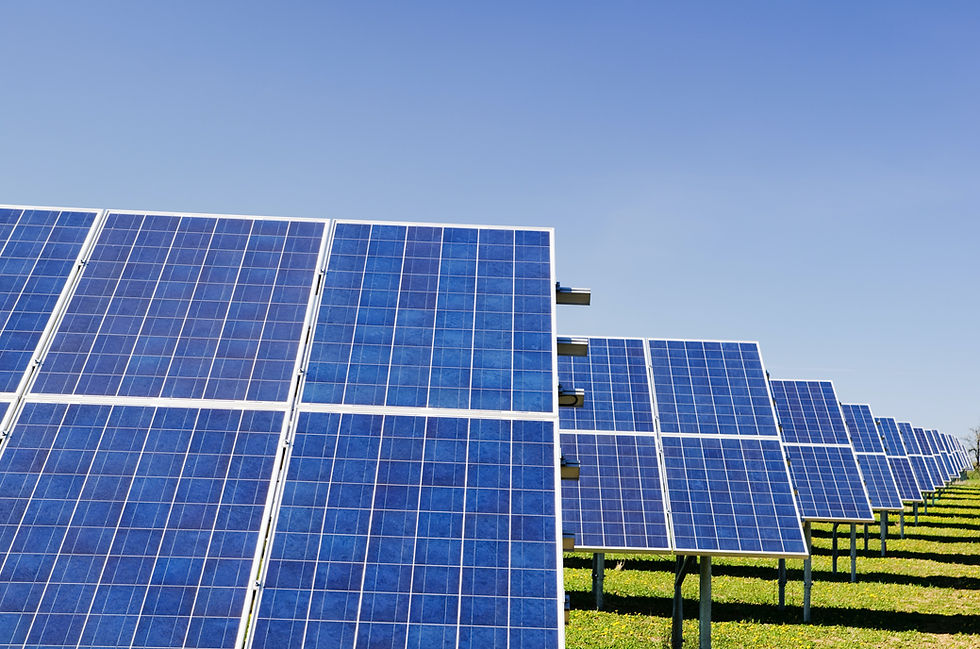
A. Importance of Accurate Energy Forecasting
Accurate energy forecasting is crucial for efficient grid management and ensuring a reliable energy supply. By analyzing historical and real-time data, AI and ML can significantly improve forecasting accuracy for renewable energy production.
B. AI in Action
Numerous renewable energy facilities now employ AI algorithms to optimize energy production based on weather forecasts, demand projections, and other relevant factors. This level of optimization ensures a more reliable energy supply and helps in reducing energy waste.
C. Advantages
Improved energy forecasting and optimization lead to better grid management, reduced energy waste, and a more reliable renewable energy supply, which are critical for the widespread adoption and success of renewable energy technologies.
IV. Automated Energy Trading and Blockchain
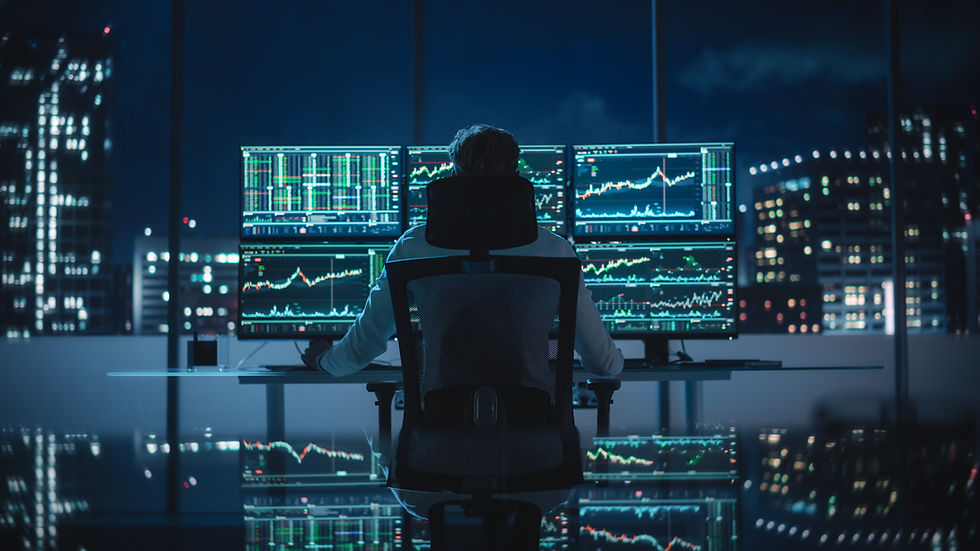
A. Overview of Automated Energy Trading
Automated energy trading platforms enable real-time buying and selling of energy, ensuring that excess energy does not go to waste. AI and ML algorithms play a crucial role in these platforms by analyzing market trends, demand-supply dynamics, and price fluctuations to facilitate efficient energy transactions.
B. Role of AI and Blockchain
AI, combined with blockchain technology, can create transparent, secure, and efficient platforms for energy transactions. Blockchain ensures a transparent and immutable record of all transactions, while AI optimizes trading decisions to ensure the best possible outcomes for both buyers and sellers.
C. Case Study: Peer-to-peer Energy Trading Platforms
Peer-to-peer (P2P) energy trading platforms, such as those powered by Power Ledger or LO3 Energy, allow prosumers to sell excess energy directly to their neighbors. AI algorithms help in optimizing the trading process, ensuring fair prices and efficient matching of buyers and sellers.
V. Enhancing Renewable Energy Design and Deployment
A. AI in Design
AI and ML algorithms can significantly contribute to the design phase of renewable energy systems. For instance, they can optimize the layout of solar panels or wind turbines to maximize energy capture based on geographic and meteorological data.
B. AI in Deployment
In the deployment phase, AI can help in managing smart grids and microgrids, ensuring that renewable energy is distributed efficiently. Furthermore, AI-driven analytics can provide insights into energy consumption patterns, helping utilities and end-users to manage energy more efficiently.
C. Benefits
Increased energy production, improved efficiency, and enhanced community resilience are among the numerous benefits that AI brings to the design and deployment of renewable energy systems. By optimizing the deployment of renewable assets and ensuring efficient energy distribution, AI contributes to creating a more sustainable and resilient energy infrastructure.
VI. Overcoming Challenges: Data Privacy and Cybersecurity
A. Discussing Potential Risks
As with any technology, the integration of AI in renewable energy systems comes with potential risks, particularly concerning data privacy and cybersecurity. The vast amount of data collected and analyzed by AI algorithms necessitates robust security measures to prevent unauthorized access and ensure data integrity.
B. Strategies for Ensuring Cybersecurity
Implementing stringent cybersecurity measures, adopting best practices, and ensuring compliance with relevant regulations are crucial steps in mitigating the risks associated with data privacy and cybersecurity in AI-driven renewable energy systems.
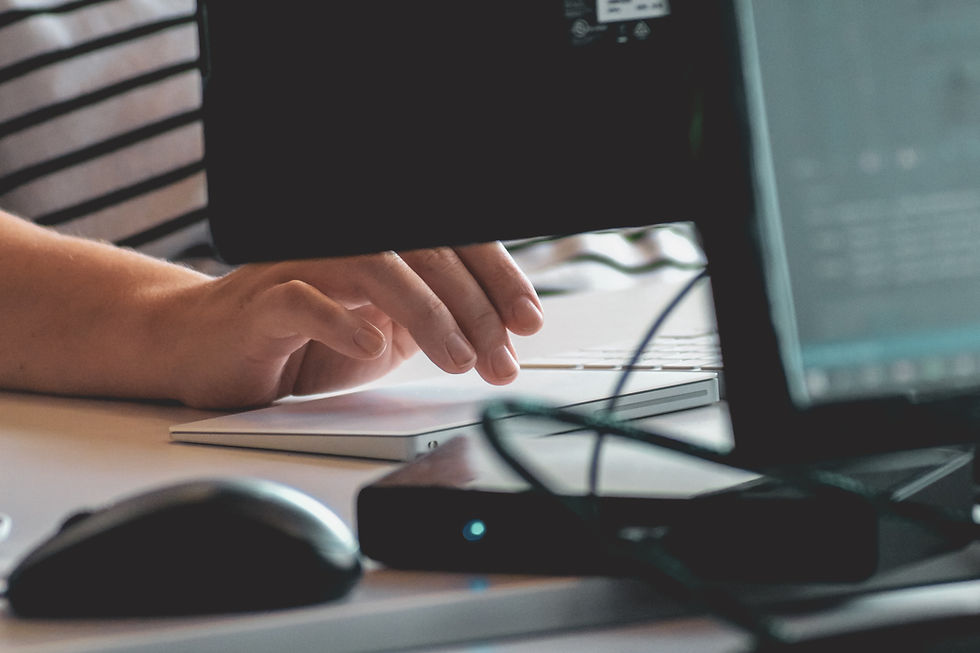
VII. Future Outlook: The Symbiosis of AI and Renewable Energy
A. Discussing Ongoing Research and Future Innovations
The symbiotic relationship between AI and renewable energy holds promise for ongoing research and future innovations. The continuous advancements in AI technology, coupled with the growing emphasis on renewable energy, are likely to drive further innovations, enhancing the efficiency and effectiveness of renewable energy systems.
B. The Long-term Impact
The long-term impact of this symbiotic relationship could be profound, driving a sustainable and smart energy future. The amalgamation of AI with renewable energy technologies has the potential to significantly accelerate the global transition towards a clean and sustainable energy ecosystem.
Conclusion
The integration of Artificial Intelligence and Machine Learning in renewable energy systems is paving the way for innovative solutions to some of the sector's most pressing challenges. By enhancing predictive maintenance, improving energy forecasting, facilitating automated energy trading, and optimizing design and deployment, AI and ML are playing a critical role in advancing the renewable energy sector towards a sustainable and bright future. Through continuous research and innovation, the symbiosis of AI and renewable energy holds the promise of a smart, efficient, and sustainable global energy landscape.
Latton Holding & AI in Renewable energy
Are you inspired by the endless possibilities of AI in renewable energy? Do you have a project or an idea that could benefit from expert insights and collaborative effort? At Latton Holding, we're passionate about exploring innovative solutions in the renewable energy sector. We invite you to Contact Us and get in touch with us to discover how we can work together towards a sustainable energy future.
Comments